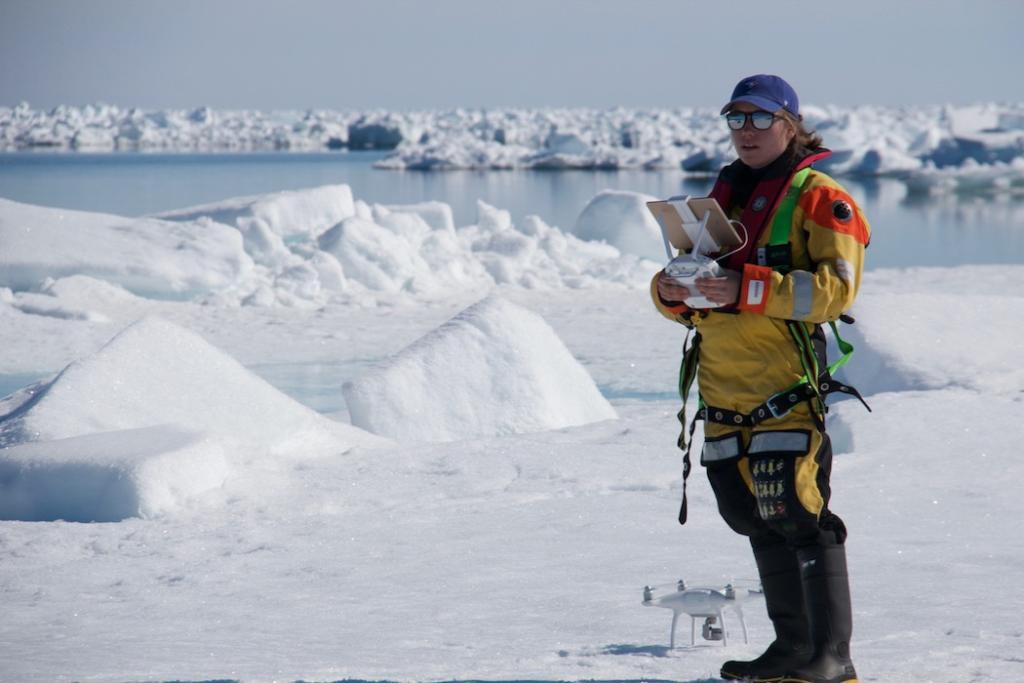
Data and Resources
Raw data
-
Churchill Estuary - Madison Harasyn, David BarberJPEG
Taken on June 13, 2018 in the Churchill Estuary as part of the Beluga Boat...
-
Churchill EstuaryJPEG
Taken on April 24, 2019 in the Churchill Estuary as part of the Churchill...
-
Churchill Estuary - Ice Floes 1JPEG
Taken on June 20, 2018 in the Churchill Estuary as part of the Churchill...
-
Churchill Estuary - Ice Floes 2JPEG
Taken on June 22, 2018 in the Churchill Estuary as part of the Churchill...
-
Churchill Estuary - Ice Floes 3JPEG
Taken on June 8, 2018 in the Churchill Estuary as part of the Churchill...
-
Churchill Estuary - Madison Harasyn and Drone JPEG
Taken on June 4, 2018 in the Churchill Estuary as part of the Churchill...
-
Churchill Estuary - Boat JPEG
Taken on June 20, 2018 in the Churchill Estuary as part of the Churchill...
-
Churchill Beluga Boat Study drone video 2019 - 1MP4
Drone footage of beluga whales and boats in the Churchill Estuary as part of...
-
Churchill Beluga Boat Study drone video 2019 - 2MP4
Drone footage of beluga whales and boats in the Churchill Estuary as part of...
-
Churchill Beluga Boat Study drone video 2019 - 3MP4
Drone footage of beluga whales and boats in the Churchill Estuary as part of...
-
Churchill Beluga Boat Study drone video 2019 - 4MP4
Drone footage of beluga whales and boats in the Churchill Estuary as part of...
-
Churchill Beluga Boat Study drone video 2019 - 5MP4
Drone footage of beluga whales and boats in the Churchill Estuary as part of...
-
Churchill Beluga Boat Study drone video 2019 - 6MP4
Drone footage of beluga whales and boats in the Churchill Estuary as part of...
-
Churchill Beluga Boat Study drone video 2019 - 7MP4
Drone footage of beluga whales and boats in the Churchill Estuary as part of...
-
Churchill Beluga Boat Study drone video 2019 - 8MP4
Drone footage of beluga whales and boats in the Churchill Estuary as part of...
Metadata
Field | Value |
---|---|
Dataset Name | Churchill Beluga Boat Drone Imagery |
Dataset General Type | imagery |
Dataset Type | Dataset |
Dataset Level | 1.1-Quality Controlled Data |
Program Website | |
Keyword Vocabulary | Polar Data Catalogue, Global Change Master Directory |
Keyword Vocabulary URL | https://www.polardata.ca/pdcinput/public/keywordlibrary ; https://gcmd.earthdata.nasa.gov/KeywordViewer/scheme/all?gtm_scheme=all |
Theme |
Freshwater
Marine Remote Sensing |
Dataset Status | Complete |
Maintenance and Update Frequency | Not planned |
Dataset Last Revision Date | 2019-08-09 |
Dataset DOI | 10.34992/sgs5-yw58 |
Metadata Creation Date | 2025 |
Publisher | CanWIN |
Field | Value |
---|---|
Dataset Collection Start Date | 2019-07-28 |
Dataset Collection End Date | 2019-08-09 |
Spatial regions | Churchill |
Spatial extent West Bound Longitude | -94.3257662171 |
Spatial extent East Bound Longitude | -94.0706395086 |
Spatial extent South Bound Latitude | 58.6686784698 |
Spatial extent North Bound Latitude | 58.8195795207 |
Field | Value |
---|---|
Sample Collection | |
Activity Collection Type | Field Measurement |
Preferred citation | |
Analytical Instrument | |
Analytical Method |
Field | Value |
---|---|
License Name | Creative Commons Attribution-NonCommercial-ShareAlike 4.0 International |
Licence Type | Open |
Embargo Date | |
Licence URL | https://spdx.org/licenses |
Terms of Access | CanWIN datasets are licensed individually, however most are licensed under the Creative Commons Attribution 4.0 International (CC BY 4.0) Public License. Details for the licence applied can be found using the Licence URL link provided with each dataset. By using data and information provided on this site you accept the terms and conditions of the License. Unless otherwise specified, the license grants the rights to the public to use and share the data and results derived therefrom as long as the proper acknowledgment is given to the data licensor (citation), that any alteration to the data is clearly indicated, and that a link to the original data and the license is made available. |
Terms of Use | By accessing this data you agree to CanWIN's Terms of Use. |
Field | Value |
---|---|
Dataset Authors |
|
Contributors | |
Project Data Curator | Chan, Wayne |
Project Data Curator email | wayne.chan@umanitoba.ca |
Project Data Curator Affiliation | Centre for Earth Observation Science - University of Manitoba |
Awards |
Field | Value |
---|---|
Related Resources |
Field | Value |
---|---|
Publications |
|